Tomaso A. Poggio
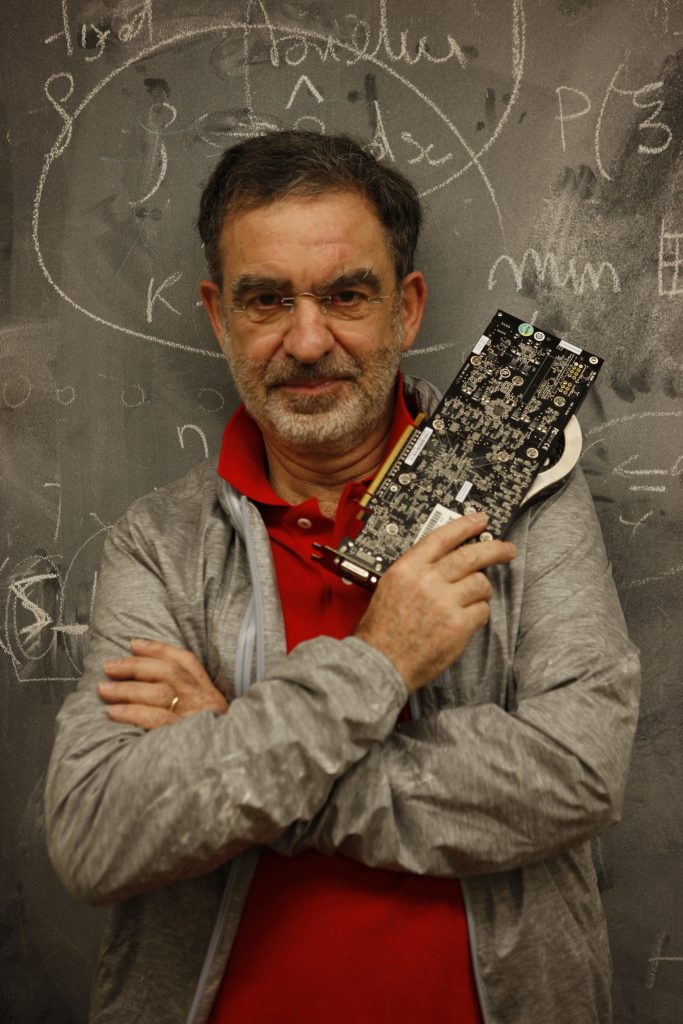
Tomaso A. Poggio is a physicist whose research has always been between brains and computers. He is now focused on the mathematics of deep learning and on the computational neuroscience of the visual cortex.
He is the Eugene McDermott Professor in the Dept. of Brain & Cognitive Sciences at MIT and the director of the NSF Center for Brains, Minds, and Machines at MIT. Among other awards, he received the 2014 Swartz Prize for Theoretical and Computational Neuroscience and the IEEE 2017 Azriel Rosenfeld Lifetime Achievement Award.
A former Corporate Fellow of Thinking Machines Corporation, a former director of PHZ Capital Partners, Inc. and of Mobileye, he was involved in starting, or investing in, several other high tech companies including Arris Pharmaceutical, nFX, Imagen, Digital Persona, Deep Mind, and Orcam.
Title: Science and Engineering of Intelligence
César A. Hidalgo
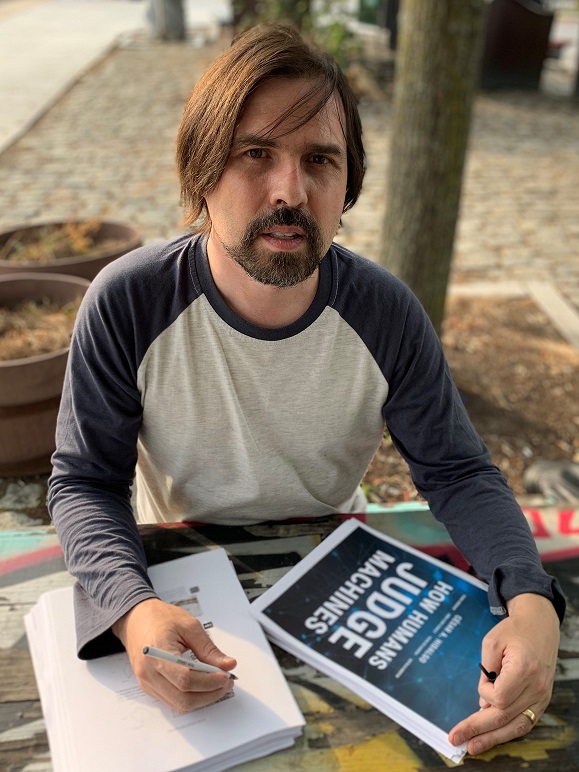
César A. Hidalgo is a Chilean-Spanish-American scholar known for his contributions to economic complexity, data visualization, and applied artificial intelligence.
Hidalgo currently holds a Chair at the Artificial and Natural Intelligence Institute (ANITI) at the University of Toulouse. He is also an Honorary Professor at the University of Manchester and a Visiting Professor at Harvard’s School of Engineering and Applied Sciences. Between 2010 and 2019 Hidalgo led MIT’s Collective Learning group, climbing through the ranks from Assistant to Associate Professor. Prior to working at MIT, Hidalgo was a research fellow at Harvard’s Kennedy School of Government. Hidalgo is also a founder of Datawheel, an award-winning company specialized in the creation of data distribution and visualization systems. He holds a Ph.D. in Physics from the University of Notre Dame and a Bachelor in Physics from Universidad Católica de Chile.
Hidalgo’s contributions have been recognized with numerous awards, including the 2018 Lagrange Prize and three Webby Awards. Hidalgo is also the author of three books: Why Information Grows (Basic Books, 2015), The Atlas of Economic Complexity (MIT Press, 2014), and How Humans Judge Machines (MIT Press, 2021).
Title: How Humans Judge Machines
Abstract: How would you feel about losing your job to a machine? How about a tsunami alert system that fails? Would you react differently to acts of discrimination performed by a machine or a human? How about public surveillance?
How Humans Judge Machines compares people’s reactions to actions performed by humans and machines. Using data collected in dozens of experiments, this book reveals the biases that permeate human-machine interactions.
Are there conditions in which we judge machines unfairly? Is our judgment of machines affected by the moral dimensions of a scenario? Is our judgment of machines correlated with demographic factors, such as education or gender?
Hidalgo and colleagues use hard science to take on these pressing technological questions. Using randomized experiments, they create revealing counterfactuals and build statistical models to explain how people judge A.I., and whether we do it fairly or not. Through original research, they bring us one step closer to understanding the ethical consequences of artificial intelligence. How Humans Judge Machines can be read for free at https://www.judgingmachines.com/
Marianne Huchard
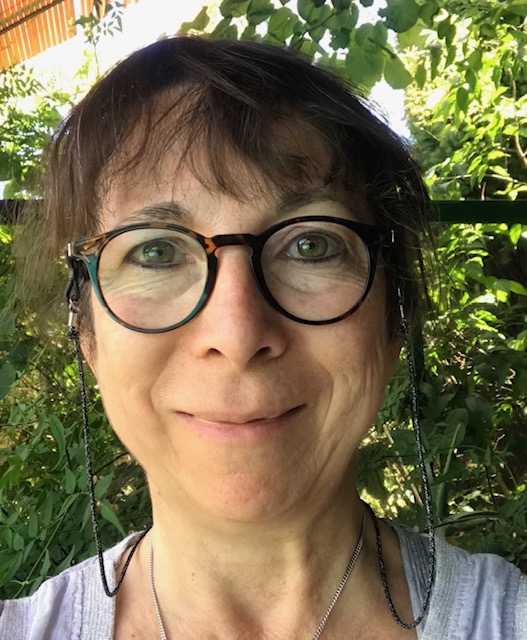
Marianne Huchard is a Full Professor of Computer Science at the University of Montpellier since 2004, where she teaches courses in knowledge engineering and software engineering. She develops her research at LIRMM (Laboratory of Informatics, Robotics, and Microelectronics at Montpellier). She obtained a Ph.D. in Computer Science in 1992, during which she investigated algorithmic questions connected to the management of multiple inheritances in various object-oriented programming languages.
She is leading research work in Formal Concept Analysis (FCA) for more than 25 years. She is recognized by the international community being Program Chair of CLA 2016 and she is regularly a member of the program committees of Concept Lattices and their Applications Conference (CLA) and International Conference on Formal Concept Analysis (ICFCA).
She contributed to various aspects of FCA: efficient algorithms; relational concept analysis (RCA), a framework that extends FCA to multi-relational datasets; the connection between RCA and other formalisms, such as propositionalization or description logics; methodology and application of FCA to several domains, including environmental datasets, ontology engineering, and as well software engineering driven by knowledge engineering and FCA.
Title: Wake the Concept Lattice in You!
Abstract: Partial orders and lattices play a major role in the scientific method, especially for ordinal and nominal data classification and comparison. Lattice theory has been developed for many years, with seminal works by R. Wille, B. Ganter, M. Barbut, B. Monjardet, and many other researchers. Strong connections with philosophy, logic, linguistics, and data science have been investigated over the years. 2022 is the 40th anniversary of the foundational paper of R. Wille “Restructuring Lattice Theory: An Approach based on Hierarchies of Concepts”, which paved the way for the dissemination of Formal Concept Analysis (FCA). FCA galaxy, including Galois connections, concept lattices, and implication bases, provides a versatile and very powerful toolbox for addressing complex knowledge extraction and exploration. It has been expanded into a large range of approaches for dealing with numerical and interval data, temporal and spatial data, sequences, graphs, or multi-relational data. Patterns structures, Fuzzy FCA, Graph-FCA, or Relational Concept Analysis are examples of such extensions. In this talk, I survey FCA from its origins, recalling key milestones. I report typical and successful applications in social sciences, life science, ontology engineering, and software engineering. I also bring to the light the existing and potential combinations with other data science approaches in the search for efficient Knowledge Discovery approaches and services.
Andrzej Skowron
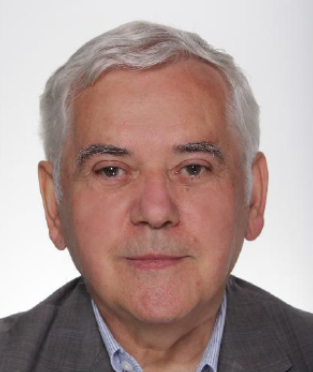
ECCAI and IRSS Fellow, Member EU Academy of Sciences, received the Ph. D. and D. Sci. (habilitation) from the University of Warsaw in Poland. In 1991 he received the Scientific Title of Professor. He is a Full Professor in the Systems Research Institute, Polish Academy of Sciences as well as in the Digital Research Center of Cardinal Stefan Wyszyński University in Warsaw. He is an Emeritus Professor in the Faculty of Mathematics, Computer Science, and Mechanics at the University of Warsaw. Andrzej Skowron is the (co)author of more than 500 scientific publications and edited books or volumes of conference proceedings. His areas of expertise include reasoning with incomplete information, approximate reasoning, soft computing methods and applications, rough sets, (interactive) granular computing, intelligent systems, knowledge discovery and data mining, decision support systems, adaptive and autonomous systems, perception-based computing. He was the supervisor of more than 20 Ph.D. Theses. In the period 1995-2009, he was the Editor-in-Chief of Fundamenta Informaticae journal. He is on the Editorial Boards of many other international journals. Andrzej Skowron was the President of the International Rough Set Society from 1996 to 2000. He has delivered numerous invited talks at international conferences. He was serving as (co-)program chair or PC member of more than 200 international conferences. He was involved in numerous research and commercial projects including dialog-based search engine (Nutech), fraud detection for Bank of America (Nutech), logistic project for General Motors (Nutech), algorithmic trading (Adgam), control of UAV (Linköping University), and medical decision support (e.g., in Polish-American Pediatric Clinic in Cracow). Andrzej Skowron was on the ICI Thomson Reuters/ Clarivate Analytics lists of the most cited researchers in Computer Science (globally) in 2012, 2016, and 2017.
Title: Perceptual Rough Set Approach in Interactive Granular Computing
Abstract: The current research on Interactive Granular Computing (IGrC), as the basis for designing Intelligent Systems (IS’s), is pointing out, in particular the need for changes in the modeling of rough sets. For example, rough sets are defined on the basis of given decision systems being purely mathematical objects. In this way, rough sets are completely embedded in the “mathematical manifold” using the terminology of Edmund Husserl, founder of phenomenology. However, (vague) concepts (or classifications) to be approximated are embedded in the real world where humans are using them, and from this physical world, their semantics should be perceived by IS’s.
The behavior of Intelligent Systems (IS’s) is based on complex granules (c-granules) and informational granules (ic-granules). Control of c-granule is making it possible to link the abstract and physical worlds. In IGrC, the perception of real situations (objects) provided by control of c-granules creates the base for the approximation of concepts by c-granules. One should be aware that physical situations (objects) can’t be omitted in perception. Hence, instead of pure mathematical objects, such as functions or relations, some non-pure mathematical constructs, like ic-granules, should be also used in the modeling of c-granule control on which the design of IS’s is proposed to be grounded. Partial information about the perceived situations is used by control of c-granules as input for approximation of concepts. However, they can be only treated as temporary models of concepts. These approximations should be continuously adapted by the relevant strategies according to changes in the perceived data recorded by c-granule. Before the control of c-granule is constructing approximation of concepts it gradually strives to better understand the perceived situations in order to make the right decision regarding construction of the concept approximations, taking into account different constraints concerning, e.g., time or other resources. Control of c-granule should provide mechanisms for deciding how c-granule should behave in perceiving the situations to obtain the relevant data, in particular by answering queries related to what, where, when, how, etc. to do. On the basis of perceived data by c-granule approximations of concepts are constructed and adapted according to observed changes in perceived data.
An attempt to link rough sets and IGrC for developing the perceptual rough set approach used in designing of IS’s, requires introducing changes in definitions of the basic concepts of the existing rough set approach. In particular, this concerns the definition of attributes in information systems (decision systems). Attributes are now grounded in the physical world and their values are perceived using constructs that are not purely mathematical functions as it is in the existing rough set approach. These constructs are called ic-granules in IGrC. They are, like physical pointers, linking the abstract objects and the physical objects from the real-physical world making it possible to perceive the relevant fragments of the current situation in the physical world. The mentioned fragments are pointed out using formal specifications of spatio-temporal windows from the abstract layers of ic-granules. The semantics of ic-granules is defined by implementation in the physical world of formal transformations of ic-granules (from the abstract layers of ic-granules). This is realized by the implementation module of c-granule. Data perceived in informational layers of ic-granules generated after implementation of submitted by control transformations of ic-granules are used to define values of attributes. Hence, the values of attributes are now obtained as the result of the perception of features of physical objects and their interactions from the relevant to the attributes configuration of ic-granules generated by control of c-granule.
In the presented approach, IGrC creates the basis for dynamically changing reasoning constructions, used for approximation of concepts (classifications) in IS’s. The required reasoning methods are far richer than nowadays used in constructing the rough set-based approximations of concepts.